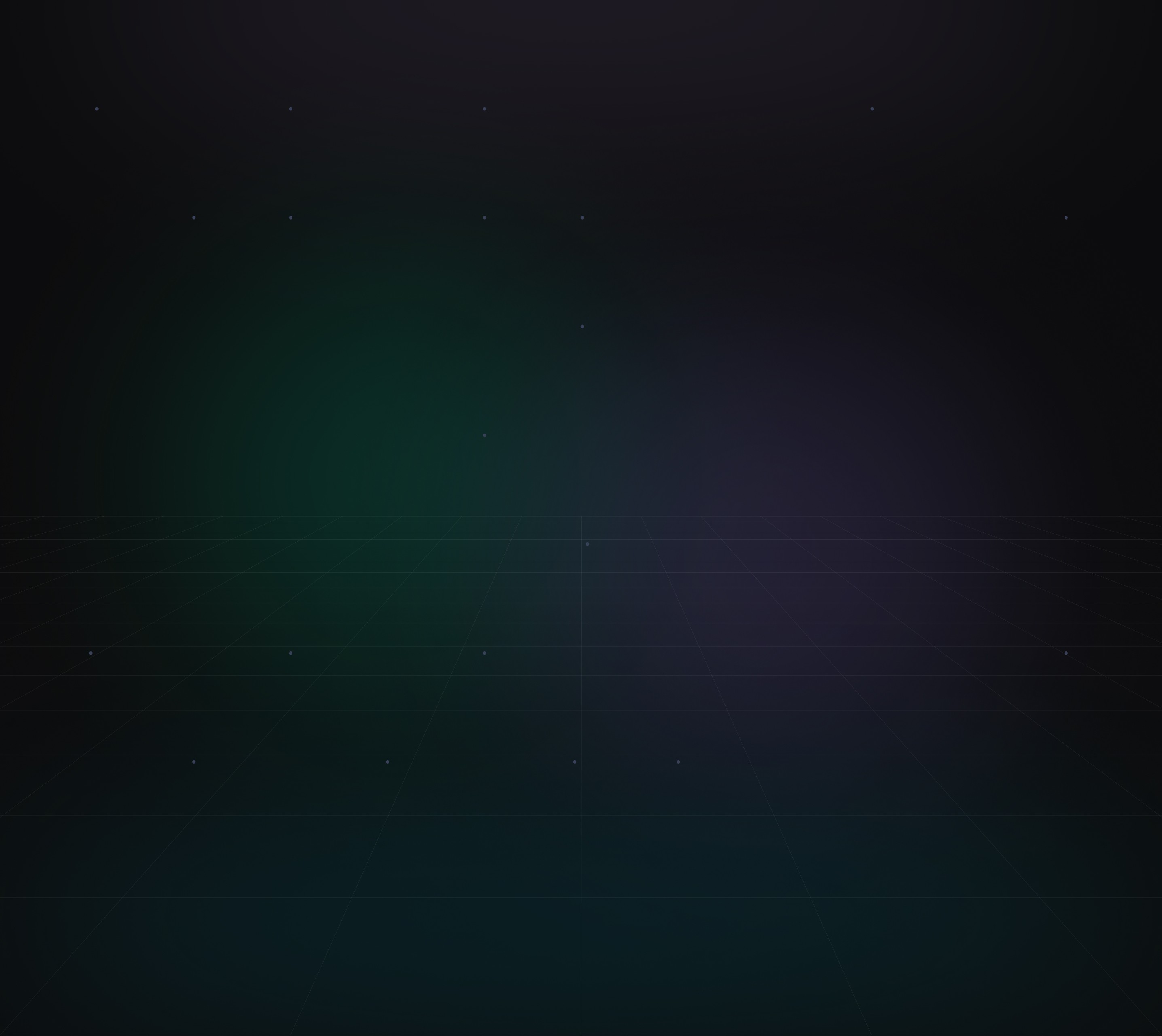

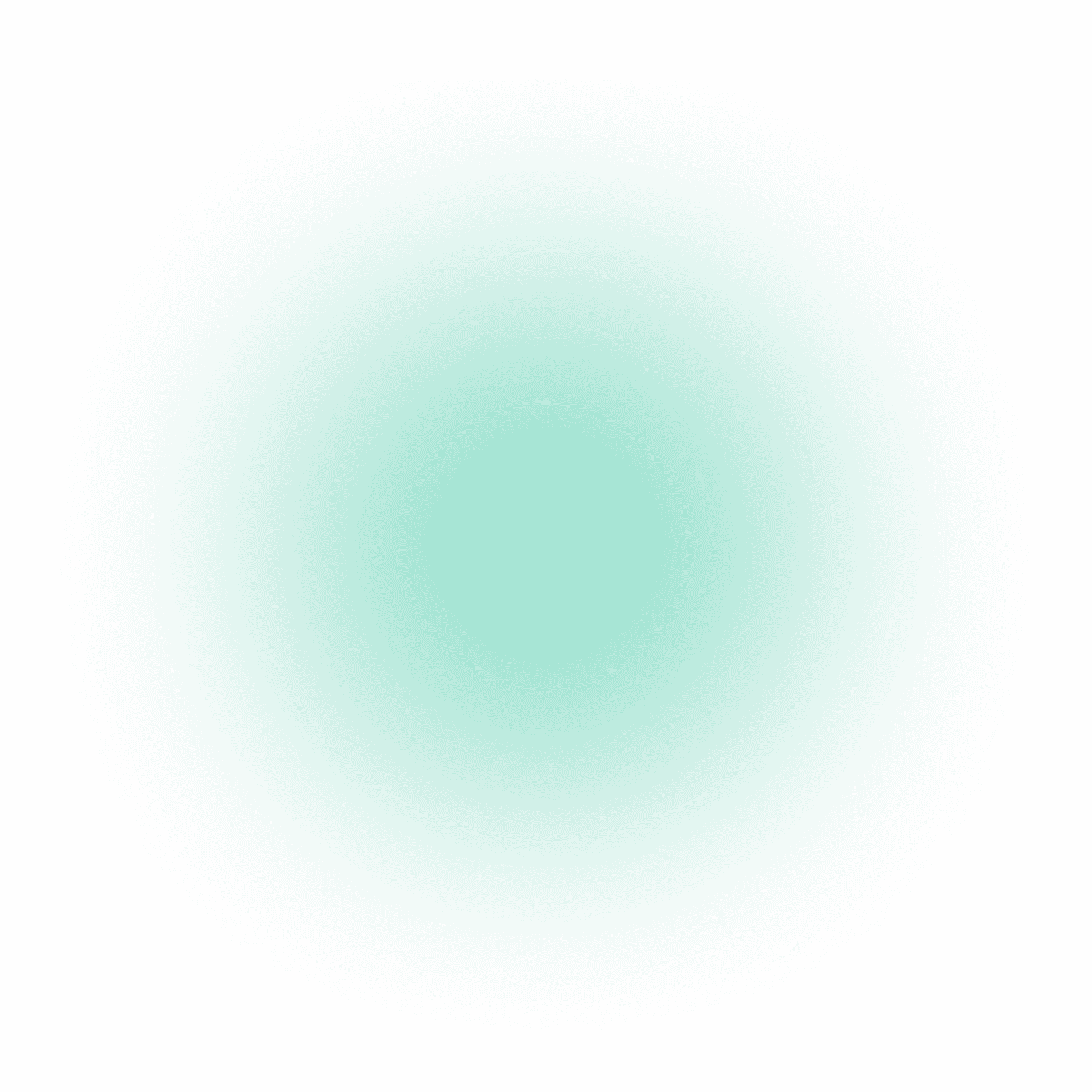

Author
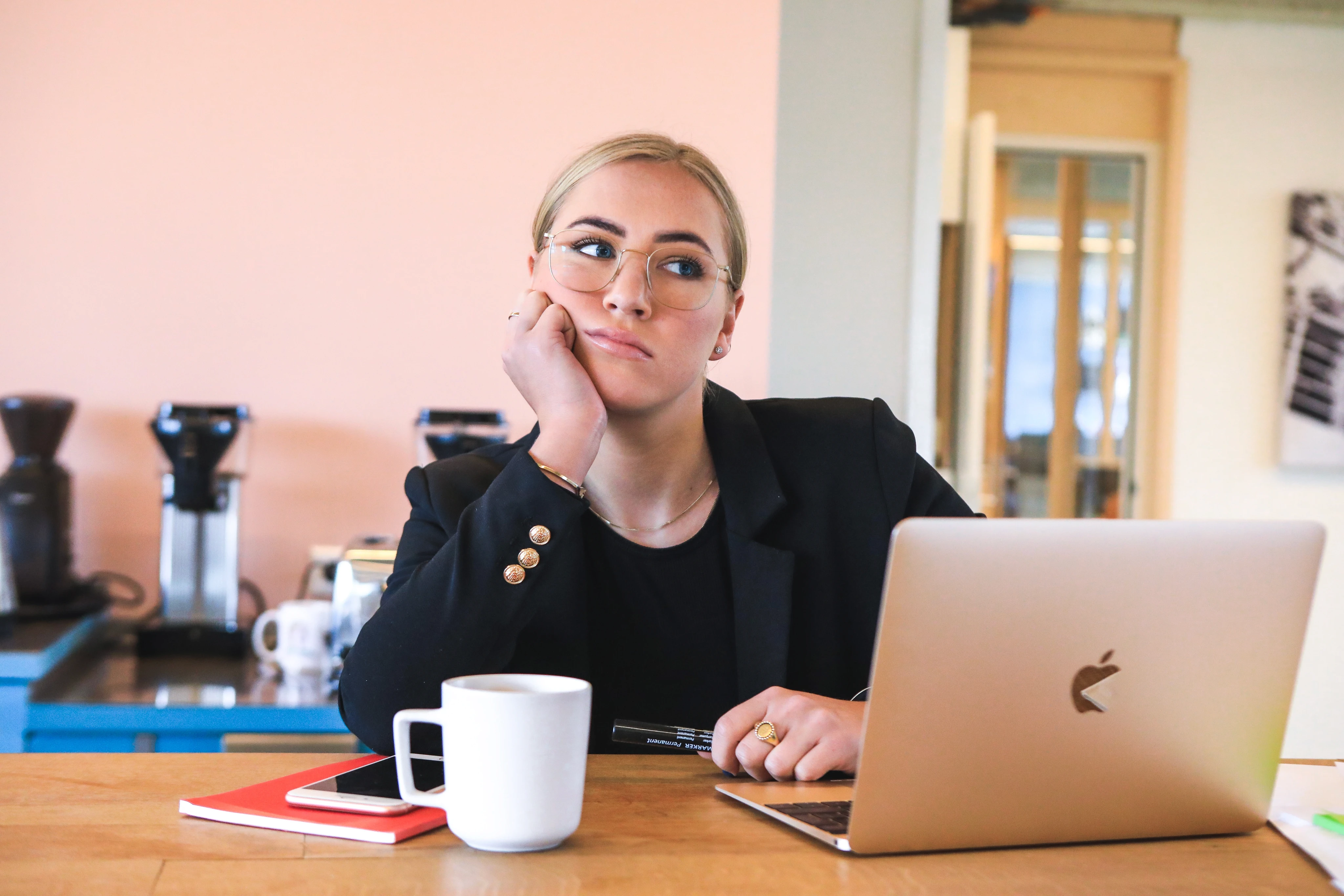
Nora Sellayemi
Jun 6, 2023
Introduction
Speech recognition technology has come a long way, revolutionizing the way we interact with machines and enabling various applications, from virtual assistants to transcription services. As speech recognition models continue to advance, it becomes essential to evaluate their performance accurately. In this comprehensive guide, we will walk you through the key steps to evaluate speech recognition models effectively, ensuring you choose the best model for your specific needs.
Accuracy and Word Error Rate (WER)
Accuracy and Word Error Rate (WER) are fundamental metrics for evaluating speech recognition models. Accuracy measures the percentage of correctly transcribed words, while WER calculates the proportion of words that were inaccurately recognized. Evaluating these metrics helps you gauge the model's overall performance and identify areas for improvement.
Language and Acoustic Model Quality
Speech recognition models consist of two main components: the language model, which represents the grammar and syntax of the language, and the acoustic model, which maps audio features to phonetic representations. Evaluating the quality of these models individually allows you to pinpoint weaknesses and optimize them accordingly.
Testing with Diverse Datasets
To assess the robustness of a speech recognition model, it's crucial to test it with diverse datasets. Use a mix of clean and noisy audio, different accents, and various speaking styles. Evaluating the model's performance across these datasets will give you a better understanding of its real-world applicability.
Confidence Measures
Confidence measures indicate the model's certainty in its transcriptions. A reliable speech recognition model should provide confidence scores for each word or transcription. Analyzing these scores allows you to filter out low-confidence predictions and increase the overall accuracy of the system.
Leveraging Perplexity
Perplexity is a metric commonly used in language modeling. It measures how well a language model predicts unseen data. Evaluating perplexity helps determine how well the model generalizes to new inputs, ensuring its effectiveness in real-world scenarios.
End-to-End vs. Hybrid Models
Speech recognition models can be categorized as end-to-end or hybrid models. End-to-end models directly convert audio to text, while hybrid models consist of multiple components, such as ASR (Automatic Speech Recognition) and NLU (Natural Language Understanding). Evaluating the trade-offs between these two approaches is crucial in choosing the right model for your application.
Conclusion
Evaluating speech recognition models is a multi-faceted process that combines quantitative metrics and qualitative user feedback. From accuracy and WER to language and acoustic model quality, each aspect contributes to determining a model's performance and suitability for real-world applications.